1. Recurrent Gamma란?
이전시간에 한국어로 파인튜닝한 gemma 2b 버전을 사용했었는데 프롬프트를 넣고 사용해 보니 영 기능이 시원치 않았다. 🤗huggingface의 google 페이지를 들어가니 recurrent gemma가 나왔더라.
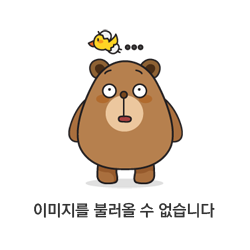
구글 홈페이지 들어가서 검색해 보니 아래와 같은 장점이 있더라
RecurrentGemma | Google for Developers
RecurrentGemma | Google for Developers
생성 및 조정 예시는 Gemma Cookbook 저장소를 확인하세요. 자세히 알아보기 이 페이지는 Cloud Translation API를 통해 번역되었습니다. 의견 보내기 컬렉션을 사용해 정리하기 내 환경설정을 기준으로
ai.google.dev
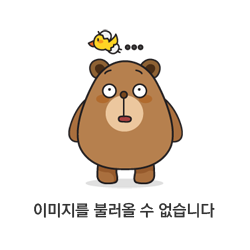
오호라... 이 모델로 하기로 결심했다. 단, 이 모델을 사용하기 위해선 🤗huggingface 의 Access Token이 있어야 한다. gemmatest라는 토큰을 하나 만들었다.
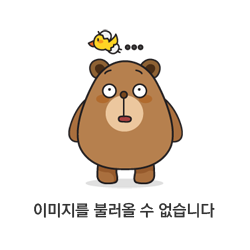
2. Recurrent Gamma 모델 구조 살펴보기
아래와 같이 주피터 노트북을 실행하여 모델 구조를 살펴보자.
import os
from tqdm.auto import tqdm
import torch
from transformers import AutoTokenizer, AutoModelForCausalLM
os.environ["HF_TOKEN"] = 'your hf token...'
model_path = "google/recurrentgemma-2b-it"
model = AutoModelForCausalLM.from_pretrained(model_path, device_map='cuda')
tokenizer = AutoTokenizer.from_pretrained(model_path, add_special_tokens=True)
model
모델 구조는 아래와 같다.
RecurrentGemmaForCausalLM(
(model): RecurrentGemmaModel(
(embed_tokens): Embedding(256000, 2560, padding_idx=0)
(layers): ModuleList(
(0-1): 2 x RecurrentGemmaDecoderLayer(
(temporal_pre_norm): RecurrentGemmaRMSNorm()
(temporal_block): RecurrentGemmaRecurrentBlock(
(linear_y): Linear(in_features=2560, out_features=2560, bias=True)
(linear_x): Linear(in_features=2560, out_features=2560, bias=True)
(linear_out): Linear(in_features=2560, out_features=2560, bias=True)
(conv_1d): Conv1d(2560, 2560, kernel_size=(4,), stride=(1,), padding=(3,), groups=2560)
(rg_lru): RecurrentGemmaRglru()
(act_fn): PytorchGELUTanh()
)
(channel_pre_norm): RecurrentGemmaRMSNorm()
(mlp_block): RecurrentGemmaMlp(
(gate_proj): Linear(in_features=2560, out_features=7680, bias=True)
(up_proj): Linear(in_features=2560, out_features=7680, bias=True)
(down_proj): Linear(in_features=7680, out_features=2560, bias=True)
(act_fn): PytorchGELUTanh()
)
)
(2): RecurrentGemmaDecoderLayer(
(temporal_pre_norm): RecurrentGemmaRMSNorm()
(temporal_block): RecurrentGemmaSdpaAttention(
(q_proj): Linear(in_features=2560, out_features=2560, bias=False)
(k_proj): Linear(in_features=2560, out_features=256, bias=False)
(v_proj): Linear(in_features=2560, out_features=256, bias=False)
(o_proj): Linear(in_features=2560, out_features=2560, bias=True)
(rotary_emb): RecurrentGemmaRotaryEmbedding()
)
(channel_pre_norm): RecurrentGemmaRMSNorm()
(mlp_block): RecurrentGemmaMlp(
(gate_proj): Linear(in_features=2560, out_features=7680, bias=True)
(up_proj): Linear(in_features=2560, out_features=7680, bias=True)
(down_proj): Linear(in_features=7680, out_features=2560, bias=True)
(act_fn): PytorchGELUTanh()
)
)
(3-4): 2 x RecurrentGemmaDecoderLayer(
(temporal_pre_norm): RecurrentGemmaRMSNorm()
(temporal_block): RecurrentGemmaRecurrentBlock(
(linear_y): Linear(in_features=2560, out_features=2560, bias=True)
(linear_x): Linear(in_features=2560, out_features=2560, bias=True)
(linear_out): Linear(in_features=2560, out_features=2560, bias=True)
(conv_1d): Conv1d(2560, 2560, kernel_size=(4,), stride=(1,), padding=(3,), groups=2560)
(rg_lru): RecurrentGemmaRglru()
(act_fn): PytorchGELUTanh()
)
(channel_pre_norm): RecurrentGemmaRMSNorm()
(mlp_block): RecurrentGemmaMlp(
(gate_proj): Linear(in_features=2560, out_features=7680, bias=True)
(up_proj): Linear(in_features=2560, out_features=7680, bias=True)
(down_proj): Linear(in_features=7680, out_features=2560, bias=True)
(act_fn): PytorchGELUTanh()
)
)
(5): RecurrentGemmaDecoderLayer(
(temporal_pre_norm): RecurrentGemmaRMSNorm()
(temporal_block): RecurrentGemmaSdpaAttention(
(q_proj): Linear(in_features=2560, out_features=2560, bias=False)
(k_proj): Linear(in_features=2560, out_features=256, bias=False)
(v_proj): Linear(in_features=2560, out_features=256, bias=False)
(o_proj): Linear(in_features=2560, out_features=2560, bias=True)
(rotary_emb): RecurrentGemmaRotaryEmbedding()
)
(channel_pre_norm): RecurrentGemmaRMSNorm()
(mlp_block): RecurrentGemmaMlp(
(gate_proj): Linear(in_features=2560, out_features=7680, bias=True)
(up_proj): Linear(in_features=2560, out_features=7680, bias=True)
(down_proj): Linear(in_features=7680, out_features=2560, bias=True)
(act_fn): PytorchGELUTanh()
)
)
(6-7): 2 x RecurrentGemmaDecoderLayer(
(temporal_pre_norm): RecurrentGemmaRMSNorm()
(temporal_block): RecurrentGemmaRecurrentBlock(
(linear_y): Linear(in_features=2560, out_features=2560, bias=True)
(linear_x): Linear(in_features=2560, out_features=2560, bias=True)
(linear_out): Linear(in_features=2560, out_features=2560, bias=True)
(conv_1d): Conv1d(2560, 2560, kernel_size=(4,), stride=(1,), padding=(3,), groups=2560)
(rg_lru): RecurrentGemmaRglru()
(act_fn): PytorchGELUTanh()
)
(channel_pre_norm): RecurrentGemmaRMSNorm()
(mlp_block): RecurrentGemmaMlp(
(gate_proj): Linear(in_features=2560, out_features=7680, bias=True)
(up_proj): Linear(in_features=2560, out_features=7680, bias=True)
(down_proj): Linear(in_features=7680, out_features=2560, bias=True)
(act_fn): PytorchGELUTanh()
)
)
(8): RecurrentGemmaDecoderLayer(
(temporal_pre_norm): RecurrentGemmaRMSNorm()
(temporal_block): RecurrentGemmaSdpaAttention(
(q_proj): Linear(in_features=2560, out_features=2560, bias=False)
(k_proj): Linear(in_features=2560, out_features=256, bias=False)
(v_proj): Linear(in_features=2560, out_features=256, bias=False)
(o_proj): Linear(in_features=2560, out_features=2560, bias=True)
(rotary_emb): RecurrentGemmaRotaryEmbedding()
)
(channel_pre_norm): RecurrentGemmaRMSNorm()
(mlp_block): RecurrentGemmaMlp(
(gate_proj): Linear(in_features=2560, out_features=7680, bias=True)
(up_proj): Linear(in_features=2560, out_features=7680, bias=True)
(down_proj): Linear(in_features=7680, out_features=2560, bias=True)
(act_fn): PytorchGELUTanh()
)
)
(9-10): 2 x RecurrentGemmaDecoderLayer(
(temporal_pre_norm): RecurrentGemmaRMSNorm()
(temporal_block): RecurrentGemmaRecurrentBlock(
(linear_y): Linear(in_features=2560, out_features=2560, bias=True)
(linear_x): Linear(in_features=2560, out_features=2560, bias=True)
(linear_out): Linear(in_features=2560, out_features=2560, bias=True)
(conv_1d): Conv1d(2560, 2560, kernel_size=(4,), stride=(1,), padding=(3,), groups=2560)
(rg_lru): RecurrentGemmaRglru()
(act_fn): PytorchGELUTanh()
)
(channel_pre_norm): RecurrentGemmaRMSNorm()
(mlp_block): RecurrentGemmaMlp(
(gate_proj): Linear(in_features=2560, out_features=7680, bias=True)
(up_proj): Linear(in_features=2560, out_features=7680, bias=True)
(down_proj): Linear(in_features=7680, out_features=2560, bias=True)
(act_fn): PytorchGELUTanh()
)
)
(11): RecurrentGemmaDecoderLayer(
(temporal_pre_norm): RecurrentGemmaRMSNorm()
(temporal_block): RecurrentGemmaSdpaAttention(
(q_proj): Linear(in_features=2560, out_features=2560, bias=False)
(k_proj): Linear(in_features=2560, out_features=256, bias=False)
(v_proj): Linear(in_features=2560, out_features=256, bias=False)
(o_proj): Linear(in_features=2560, out_features=2560, bias=True)
(rotary_emb): RecurrentGemmaRotaryEmbedding()
)
(channel_pre_norm): RecurrentGemmaRMSNorm()
(mlp_block): RecurrentGemmaMlp(
(gate_proj): Linear(in_features=2560, out_features=7680, bias=True)
(up_proj): Linear(in_features=2560, out_features=7680, bias=True)
(down_proj): Linear(in_features=7680, out_features=2560, bias=True)
(act_fn): PytorchGELUTanh()
)
)
(12-13): 2 x RecurrentGemmaDecoderLayer(
(temporal_pre_norm): RecurrentGemmaRMSNorm()
(temporal_block): RecurrentGemmaRecurrentBlock(
(linear_y): Linear(in_features=2560, out_features=2560, bias=True)
(linear_x): Linear(in_features=2560, out_features=2560, bias=True)
(linear_out): Linear(in_features=2560, out_features=2560, bias=True)
(conv_1d): Conv1d(2560, 2560, kernel_size=(4,), stride=(1,), padding=(3,), groups=2560)
(rg_lru): RecurrentGemmaRglru()
(act_fn): PytorchGELUTanh()
)
(channel_pre_norm): RecurrentGemmaRMSNorm()
(mlp_block): RecurrentGemmaMlp(
(gate_proj): Linear(in_features=2560, out_features=7680, bias=True)
(up_proj): Linear(in_features=2560, out_features=7680, bias=True)
(down_proj): Linear(in_features=7680, out_features=2560, bias=True)
(act_fn): PytorchGELUTanh()
)
)
(14): RecurrentGemmaDecoderLayer(
(temporal_pre_norm): RecurrentGemmaRMSNorm()
(temporal_block): RecurrentGemmaSdpaAttention(
(q_proj): Linear(in_features=2560, out_features=2560, bias=False)
(k_proj): Linear(in_features=2560, out_features=256, bias=False)
(v_proj): Linear(in_features=2560, out_features=256, bias=False)
(o_proj): Linear(in_features=2560, out_features=2560, bias=True)
(rotary_emb): RecurrentGemmaRotaryEmbedding()
)
(channel_pre_norm): RecurrentGemmaRMSNorm()
(mlp_block): RecurrentGemmaMlp(
(gate_proj): Linear(in_features=2560, out_features=7680, bias=True)
(up_proj): Linear(in_features=2560, out_features=7680, bias=True)
(down_proj): Linear(in_features=7680, out_features=2560, bias=True)
(act_fn): PytorchGELUTanh()
)
)
(15-16): 2 x RecurrentGemmaDecoderLayer(
(temporal_pre_norm): RecurrentGemmaRMSNorm()
(temporal_block): RecurrentGemmaRecurrentBlock(
(linear_y): Linear(in_features=2560, out_features=2560, bias=True)
(linear_x): Linear(in_features=2560, out_features=2560, bias=True)
(linear_out): Linear(in_features=2560, out_features=2560, bias=True)
(conv_1d): Conv1d(2560, 2560, kernel_size=(4,), stride=(1,), padding=(3,), groups=2560)
(rg_lru): RecurrentGemmaRglru()
(act_fn): PytorchGELUTanh()
)
(channel_pre_norm): RecurrentGemmaRMSNorm()
(mlp_block): RecurrentGemmaMlp(
(gate_proj): Linear(in_features=2560, out_features=7680, bias=True)
(up_proj): Linear(in_features=2560, out_features=7680, bias=True)
(down_proj): Linear(in_features=7680, out_features=2560, bias=True)
(act_fn): PytorchGELUTanh()
)
)
(17): RecurrentGemmaDecoderLayer(
(temporal_pre_norm): RecurrentGemmaRMSNorm()
(temporal_block): RecurrentGemmaSdpaAttention(
(q_proj): Linear(in_features=2560, out_features=2560, bias=False)
(k_proj): Linear(in_features=2560, out_features=256, bias=False)
(v_proj): Linear(in_features=2560, out_features=256, bias=False)
(o_proj): Linear(in_features=2560, out_features=2560, bias=True)
(rotary_emb): RecurrentGemmaRotaryEmbedding()
)
(channel_pre_norm): RecurrentGemmaRMSNorm()
(mlp_block): RecurrentGemmaMlp(
(gate_proj): Linear(in_features=2560, out_features=7680, bias=True)
(up_proj): Linear(in_features=2560, out_features=7680, bias=True)
(down_proj): Linear(in_features=7680, out_features=2560, bias=True)
(act_fn): PytorchGELUTanh()
)
)
(18-19): 2 x RecurrentGemmaDecoderLayer(
(temporal_pre_norm): RecurrentGemmaRMSNorm()
(temporal_block): RecurrentGemmaRecurrentBlock(
(linear_y): Linear(in_features=2560, out_features=2560, bias=True)
(linear_x): Linear(in_features=2560, out_features=2560, bias=True)
(linear_out): Linear(in_features=2560, out_features=2560, bias=True)
(conv_1d): Conv1d(2560, 2560, kernel_size=(4,), stride=(1,), padding=(3,), groups=2560)
(rg_lru): RecurrentGemmaRglru()
(act_fn): PytorchGELUTanh()
)
(channel_pre_norm): RecurrentGemmaRMSNorm()
(mlp_block): RecurrentGemmaMlp(
(gate_proj): Linear(in_features=2560, out_features=7680, bias=True)
(up_proj): Linear(in_features=2560, out_features=7680, bias=True)
(down_proj): Linear(in_features=7680, out_features=2560, bias=True)
(act_fn): PytorchGELUTanh()
)
)
(20): RecurrentGemmaDecoderLayer(
(temporal_pre_norm): RecurrentGemmaRMSNorm()
(temporal_block): RecurrentGemmaSdpaAttention(
(q_proj): Linear(in_features=2560, out_features=2560, bias=False)
(k_proj): Linear(in_features=2560, out_features=256, bias=False)
(v_proj): Linear(in_features=2560, out_features=256, bias=False)
(o_proj): Linear(in_features=2560, out_features=2560, bias=True)
(rotary_emb): RecurrentGemmaRotaryEmbedding()
)
(channel_pre_norm): RecurrentGemmaRMSNorm()
(mlp_block): RecurrentGemmaMlp(
(gate_proj): Linear(in_features=2560, out_features=7680, bias=True)
(up_proj): Linear(in_features=2560, out_features=7680, bias=True)
(down_proj): Linear(in_features=7680, out_features=2560, bias=True)
(act_fn): PytorchGELUTanh()
)
)
(21-22): 2 x RecurrentGemmaDecoderLayer(
(temporal_pre_norm): RecurrentGemmaRMSNorm()
(temporal_block): RecurrentGemmaRecurrentBlock(
(linear_y): Linear(in_features=2560, out_features=2560, bias=True)
(linear_x): Linear(in_features=2560, out_features=2560, bias=True)
(linear_out): Linear(in_features=2560, out_features=2560, bias=True)
(conv_1d): Conv1d(2560, 2560, kernel_size=(4,), stride=(1,), padding=(3,), groups=2560)
(rg_lru): RecurrentGemmaRglru()
(act_fn): PytorchGELUTanh()
)
(channel_pre_norm): RecurrentGemmaRMSNorm()
(mlp_block): RecurrentGemmaMlp(
(gate_proj): Linear(in_features=2560, out_features=7680, bias=True)
(up_proj): Linear(in_features=2560, out_features=7680, bias=True)
(down_proj): Linear(in_features=7680, out_features=2560, bias=True)
(act_fn): PytorchGELUTanh()
)
)
(23): RecurrentGemmaDecoderLayer(
(temporal_pre_norm): RecurrentGemmaRMSNorm()
(temporal_block): RecurrentGemmaSdpaAttention(
(q_proj): Linear(in_features=2560, out_features=2560, bias=False)
(k_proj): Linear(in_features=2560, out_features=256, bias=False)
(v_proj): Linear(in_features=2560, out_features=256, bias=False)
(o_proj): Linear(in_features=2560, out_features=2560, bias=True)
(rotary_emb): RecurrentGemmaRotaryEmbedding()
)
(channel_pre_norm): RecurrentGemmaRMSNorm()
(mlp_block): RecurrentGemmaMlp(
(gate_proj): Linear(in_features=2560, out_features=7680, bias=True)
(up_proj): Linear(in_features=2560, out_features=7680, bias=True)
(down_proj): Linear(in_features=7680, out_features=2560, bias=True)
(act_fn): PytorchGELUTanh()
)
)
(24-25): 2 x RecurrentGemmaDecoderLayer(
(temporal_pre_norm): RecurrentGemmaRMSNorm()
(temporal_block): RecurrentGemmaRecurrentBlock(
(linear_y): Linear(in_features=2560, out_features=2560, bias=True)
(linear_x): Linear(in_features=2560, out_features=2560, bias=True)
(linear_out): Linear(in_features=2560, out_features=2560, bias=True)
(conv_1d): Conv1d(2560, 2560, kernel_size=(4,), stride=(1,), padding=(3,), groups=2560)
(rg_lru): RecurrentGemmaRglru()
(act_fn): PytorchGELUTanh()
)
(channel_pre_norm): RecurrentGemmaRMSNorm()
(mlp_block): RecurrentGemmaMlp(
(gate_proj): Linear(in_features=2560, out_features=7680, bias=True)
(up_proj): Linear(in_features=2560, out_features=7680, bias=True)
(down_proj): Linear(in_features=7680, out_features=2560, bias=True)
(act_fn): PytorchGELUTanh()
)
)
)
(final_norm): RecurrentGemmaRMSNorm()
)
(lm_head): Linear(in_features=2560, out_features=256000, bias=False)
)
2b 모델임에도 다른 모델들보다 구조가 엄청난 느낌이다;;; 256000이라는 입력벡터와 출력벡터의 차원수도 눈에 띈다. 이 모델... 과연 한국어를 잘 할 수 있을까?
3. 한국어 출력 테스트
1) 기본 텍스트 입력
먼저 가볍게 시작해 보자. 프롬프트 없이 텍스트만 입력해 보자.
input_text = "제주도 1박 2일 코드를 알려줘"
input_ids = tokenizer(input_text, return_tensors="pt").to("cuda")
output = model.generate(**input_ids, max_new_tokens=200)
print(tokenizer.decode(output[0]))
<bos>제주도 1박 2일 코드를 알려줘
제주도 1박 2일의 코드는 다음과 같습니다.
- **첫째 날:**
- 오전: 제주도의 대표적인 볼거리인 쇼핑몰, 쇼핑가를 방문합니다.
- 오후: 제주도의 대표적인 해수욕장인 제주도 해수욕장을 방문합니다.
- **둘째 날:**
- 오전: 제주도의 대표적인 농장, 목장을 방문합니다.
- 오후: 제주도의 대표적인 공원, 정원을 방문합니다.<eos>
명확하게 <eos> 토큰으로 끝났다. 대표적인 볼거리인 쇼핑몰과 쇼핑가가 어디인지, 대표적인 농장과 목장, 공원, 정원은 어디인지 알려주지 않아서 아쉬웠지만 나름 쓸만? 한 느낌이다.
이전 시간에서 "현재 대한민국은" 넣었더니
<bos>현재 대한민국은 1948년 8월 15일 대한민
이런식으로 출력되었었는데 모두 gemma 2b 한국어 파인튜닝 모델이었다. 과연 이번 모델은 어떨까?
input_text = "현재 대한민국은"
input_ids = tokenizer(input_text, return_tensors="pt").to("cuda")
output = model.generate(**input_ids, max_new_tokens=200)
print(tokenizer.decode(output[0]))
<bos>현재 대한민국은 1948년에 설립된 민주주의 국가이며, 1987년에 개정된宪法을 가지고 있습니다. 이러한 점에서 대한민국은 짧은 시간에 민주주의를 구축하고, 지속적으로 발전하는 나라로 알려져 있습니다.
하지만, 대한민국은 여전히 Some of the challenges that it faces include:
* 낮은 인구 성장: 대한민국은 인구가 낮고, 성장률이 낮기 때문에 경제 활성화에 어려움을 겪고 있습니다.
* 빈곤: 대한민국은 빈곤층이 40%에 달하는 빈곤 문제가 심각합니다.
* 범죄율: 대한민국은 범죄율이 높고, 특히 암살과 불법물품 난 slinging이 심화되고 있습니다.
* 환
오! 이전보단 훨씬 낫다. 2b 모델임에도 불구하고 한국어 훈련이 괜찮게 되어있는 모양이다.
2) 프롬프트 사용을 위한 apply_chat_template 메서드 사용하기
모델마다 학습된 스페셜 토큰이라는 것이 있다. 문장의 시작, 끝, 문장 구분 여부, 분류, 마스킹 등 어떻게 문장을 이해하고 작업해야 하는지 알려주어야 한다. 이러한 스페셜 토큰을 이용하여 구성한 것이 모델이 프롬프트이다.
🤗huggingface 에서 speical_tokens_map.json 파일에 들어가면 어떤 스페셜 토큰이 사용되는지 알 수 있다.
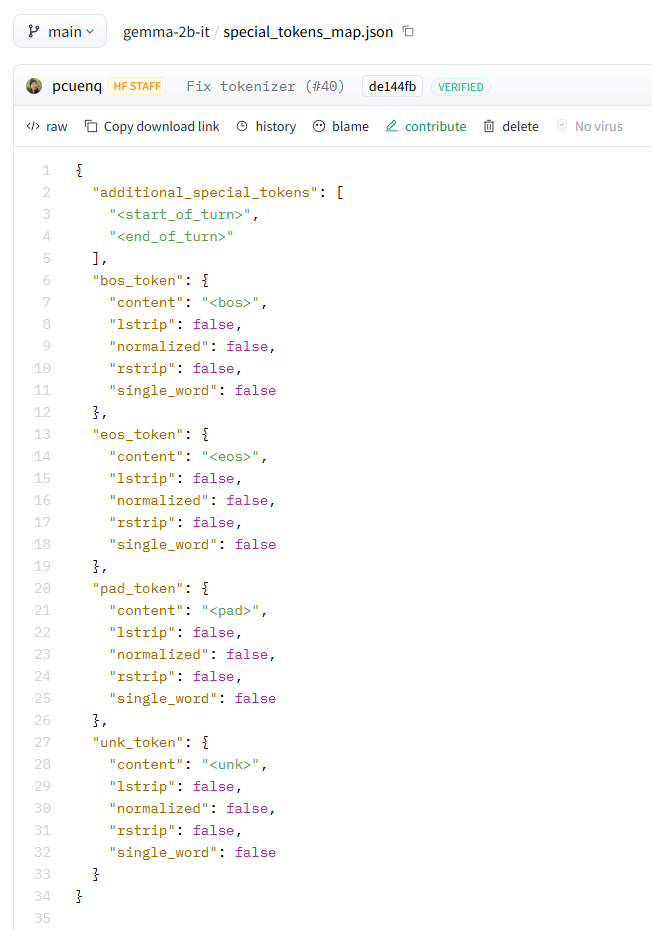
아래 구글 홈페이지에 들어가면 gemma의 프롬프트 템플릿을 상세히 알려준다.
Gemma 형식 및 시스템 안내 | Google for Developers
Gemma 형식 및 시스템 안내 | Google for Developers
생성 및 조정 예시는 Gemma Cookbook 저장소를 확인하세요. 자세히 알아보기 이 페이지는 Cloud Translation API를 통해 번역되었습니다. 의견 보내기 Gemma 형식 및 시스템 안내 컬렉션을 사용해 정리하기
ai.google.dev
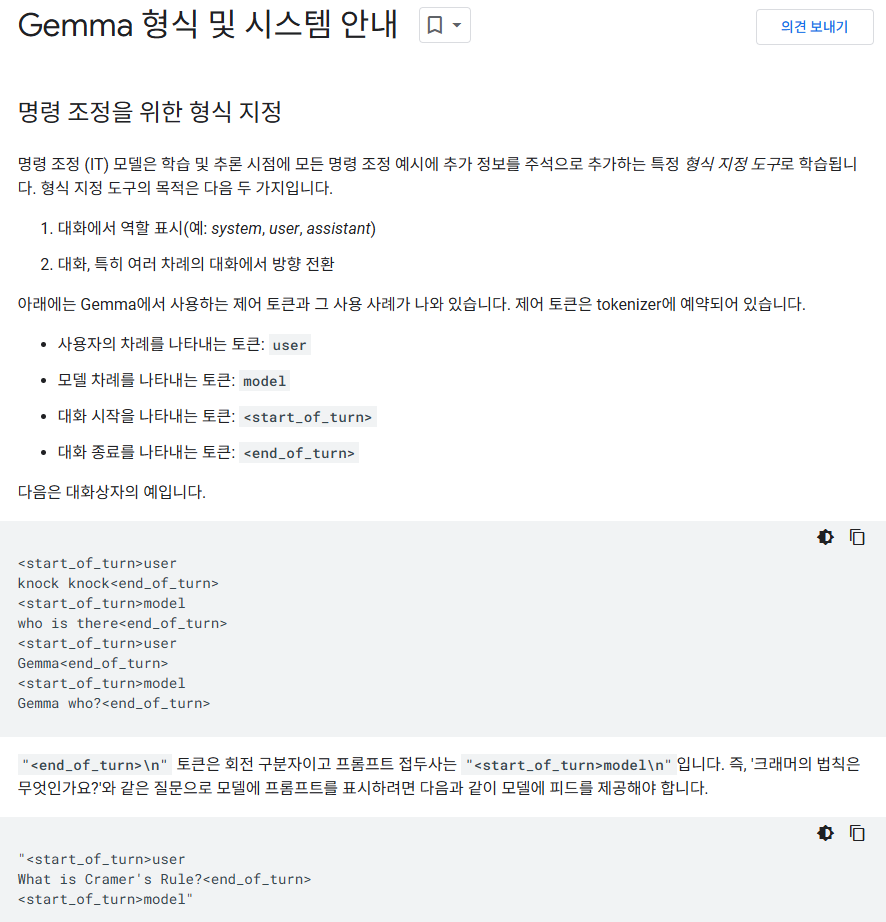
이렇게 프롬프트를 작성하기 귀찮으니까, tokenizer에 apply_chat_template 메서드에서는 아래와 같이 프롬프트 템플릿을 쉽게 사용할 수 있게 해 준다.
user, assistant, content의 역할만 정확하게 알면 비교적 쉽다. 자세한 내용은 아래 링크 참고
Templates for Chat Models (huggingface.co)
Templates for Chat Models
An increasingly common use case for LLMs is chat. In a chat context, rather than continuing a single string of text (as is the case with a standard language model), the model instead continues a conversation that consists of one or more messages, each of w
huggingface.co
이 기능을 이용하여 동요 작사 프롬프트를 입력해 보자.
doc = "다람쥐, 도토리 "
message = [
{
"role": "user",
"content": "제시된 단어를 이용하여 간단한 동요를 한 문장 작사해주세요.:{}".format(doc)
}
]
print(message)
[{'role': 'user', 'content': '제시된 단어를 이용하여 간단한 동요를 한 문장 작사해주세요.:다람쥐, 도토리 '}]
message를 apply_chat_template에 입력해 보자.
tokenized_chat = tokenizer.apply_chat_template(message, tokenize=True,
return_tensors="pt",
skip_special_tokens=False,
add_generation_prompt=False).to("cuda")
print(tokenized_chat, "\n")
print(tokenizer.decode(tokenized_chat[0]))
아래와 같이 토큰화된 것을 알 수 있다.
tensor([[ 2, 106, 1645, 108, 236939, 236569, 238602, 80289, 236770,
236791, 208134, 72494, 127149, 238335, 236511, 49697, 237526, 236791,
35191, 45980, 237199, 63806, 236417, 237138, 237014, 96673, 5672,
236039, 238912, 243469, 235269, 50316, 238772, 236432, 107, 108]],
device='cuda:0')
<bos><start_of_turn>user
제시된 단어를 이용하여 간단한 동요를 한 문장 작사해주세요.:다람쥐, 도토리<end_of_turn>
모델에 입력해보자.
output = model.generate(tokenized_chat, max_new_tokens=100)
print(tokenizer.decode(output[0]))
음 출력이 좀 아쉽다. 반복은 동요의 특징 중 하나이지만 LLM에서 반복은 제대로 된 출력을 찾지 못했다는 뜻이다.
<bos><start_of_turn>user
제시된 단어를 이용하여 간단한 동요를 한 문장 작사해주세요.:다람쥐, 도토리<end_of_turn>
다람쥐, 도토리,
밤에 밤에 밤에,
동요, 동요, 동요.<eos>
one shot 프롬프팅 기술을 사용해 보겠다. 간단한 동요 작사 방법을 하나 알려주는 것이다. 이를 위해 아래와 같이 message를 구성할 수 있다.
doc = "다람쥐, 도토리 "
message = [
{
"role": "user",
"content": "제시된 단어를 이용하여 간단한 동요를 한 문장 작사해주세요.: 토끼, 당근"
},
{
"role": "assistant",
"content": "토끼가 잠을 자다가 당근을 보았어요.\n 토기가 잠에서 깨어 당근을 찾아요."
},
{
"role": "user",
"content": "제시된 단어를 이용하여 간단한 동요를 한 문장 작사해주세요.:{}".format(doc)
}
]
print(message)
tokenized_chat = tokenizer.apply_chat_template(message, tokenize=True,
return_tensors="pt",
skip_special_tokens=False,
add_generation_prompt=False).to("cuda")
output = model.generate(tokenized_chat, max_new_tokens=100)
print(tokenizer.decode(output[0]))
출력 결과를 살펴보면 뭔가 이상하지만 제시된 양식을 지키려고 노력한 것을 알 수 있다. 그런데 다람쥐에 자꾸 밤이 왜 나오는거야? 이게 먹는 밤이여 낮과 밤이여?
<bos><start_of_turn>user
제시된 단어를 이용하여 간단한 동요를 한 문장 작사해주세요.: 토끼, 당근<end_of_turn>
<start_of_turn>model
토끼가 잠을 자다가 당근을 보았어요.
토기가 잠에서 깨어 당근을 찾아요.<end_of_turn>
<start_of_turn>user
제시된 단어를 이용하여 간단한 동요를 한 문장 작사해주세요.:다람쥐, 도토리<end_of_turn>
다람쥐가 밤을 보다가 도토리를 봤어요.
다람쥐가 밤에서 깨어 도토리를 찾아요.<eos>
3) 프롬프트 직접 구성하기
아래와 같이 프롬프트를 직접 구성할 수 있다. 차이는 없다고 봐도 무방하다.
doc = "다람쥐, 도토리"
message = [r"""<bos><start_of_turn>user
제시된 단어를 이용하여 간단한 동요를 한 문장 작사해주세요. :{}<end_of_turn>
<start_of_turn>model
<eos>""".format(doc)]
print(message)
input_ids = tokenizer(message, return_tensors="pt").to("cuda")
output = model.generate(**input_ids, max_new_tokens=100)
print(tokenizer.decode(output[0]))
<bos><bos><start_of_turn>user
제시된 단어를 이용하여 간단한 동요를 한 문장 작사해주세요. :다람쥐, 도토리<end_of_turn>
<start_of_turn>model
<eos>다람쥐, 도토리, 밤하늘을 듣고, 밤하늘을 듣고, 다람쥐, 도토리.<eos>
one shot도 아래와 같이 구성할 수 있다.
doc = "다람쥐, 도토리 "
message = [r"""<bos><start_of_turn>user
제시된 단어를 이용하여 간단한 동요를 한 문장 작사해주세요.: 토끼, 당근 <end_of_turn>
<start_of_turn>model
토끼가 잠을 자다가 당근을 보았어요.\n 토기가 잠에서 깨어 당근을 찾아요<end_of_turn>
<start_of_utrn>user
제시된 단어를 이용하여 간단한 동요를 한 문장 작사해주세요.:{}<end_of_turn>
<start_of_turn>model
<end_of_turn><eos>""".format(doc)]
print(message)
input_ids = tokenizer(message, return_tensors="pt").to("cuda")
output = model.generate(**input_ids, max_new_tokens=100)
print(tokenizer.decode(output[0]))
<bos><bos><start_of_turn>user
제시된 단어를 이용하여 간단한 동요를 한 문장 작사해주세요.: 토끼, 당근 <end_of_turn>
<start_of_turn>model
토끼가 잠을 자다가 당근을 보았어요.\n 토기가 잠에서 깨어 당근을 찾아요<end_of_turn>
<start_of_utrn>user
제시된 단어를 이용하여 간단한 동요를 한 문장 작사해주세요.:다람쥐, 도토리 <end_of_turn>
<start_of_turn>model
<end_of_turn><eos>다람쥐가 밤을 보다가 도토리를 봤어요.
다람쥐가 밤에서 깨어 도토리를 찾아요.<eos>
4) 파이프라인 이용하기
아래와 같이 파이프라인을 이용할 수 있다.
from transformers import pipeline
pipe = pipeline("text-generation", model=model, tokenizer=tokenizer, max_new_tokens=200)
output = pipe(message, do_sample=True, temperature=0.2, top_k=50, top_p=0.95, add_special_tokens=True)
print(output)
[[{'generated_text': '<bos><start_of_turn>user\n제시된 단어를 이용하여 간단한 동요를 한 문장 작사해주세요.: 토끼, 당근 <end_of_turn>\n<start_of_turn>model\n토끼가 잠을 자다가 당근을 보았어요.\\n 토기가 잠에서 깨어 당근을 찾아요<end_of_turn>\n<start_of_utrn>user\n제시된 단어를 이용하여 간단한 동요를 한 문장 작사해주세요.:다람쥐, 도토리 <end_of_turn>\n<start_of_turn>model\n<end_of_turn><eos>다람쥐가 밤을 보다가 도토리를 봤어요.\n다람쥐가 밤에서 깨어 도토리를 찾아요.'}]]
'🤗허깅페이스(Hugging Face) > 🤗트랜스포머(Transformer) 활용하기' 카테고리의 다른 글
gemma-2-2b-it 파인튜닝하기 (0) | 2024.10.11 |
---|---|
recurrent gemma 2b 훈련을 위한 데이터 준비 및 처리하기 (0) | 2024.10.03 |
Recurrent Gemma 2b와 프롬프트로 심리상담 챗봇 만들기 (0) | 2024.10.02 |
🤗huggingface LLM 개발을 위한 아나콘다 환경 구성 (0) | 2024.07.16 |
🤗 [시작하기] 1. 허깅페이스 트랜스포머 라이브러리란? (0) | 2023.08.25 |